Read blog
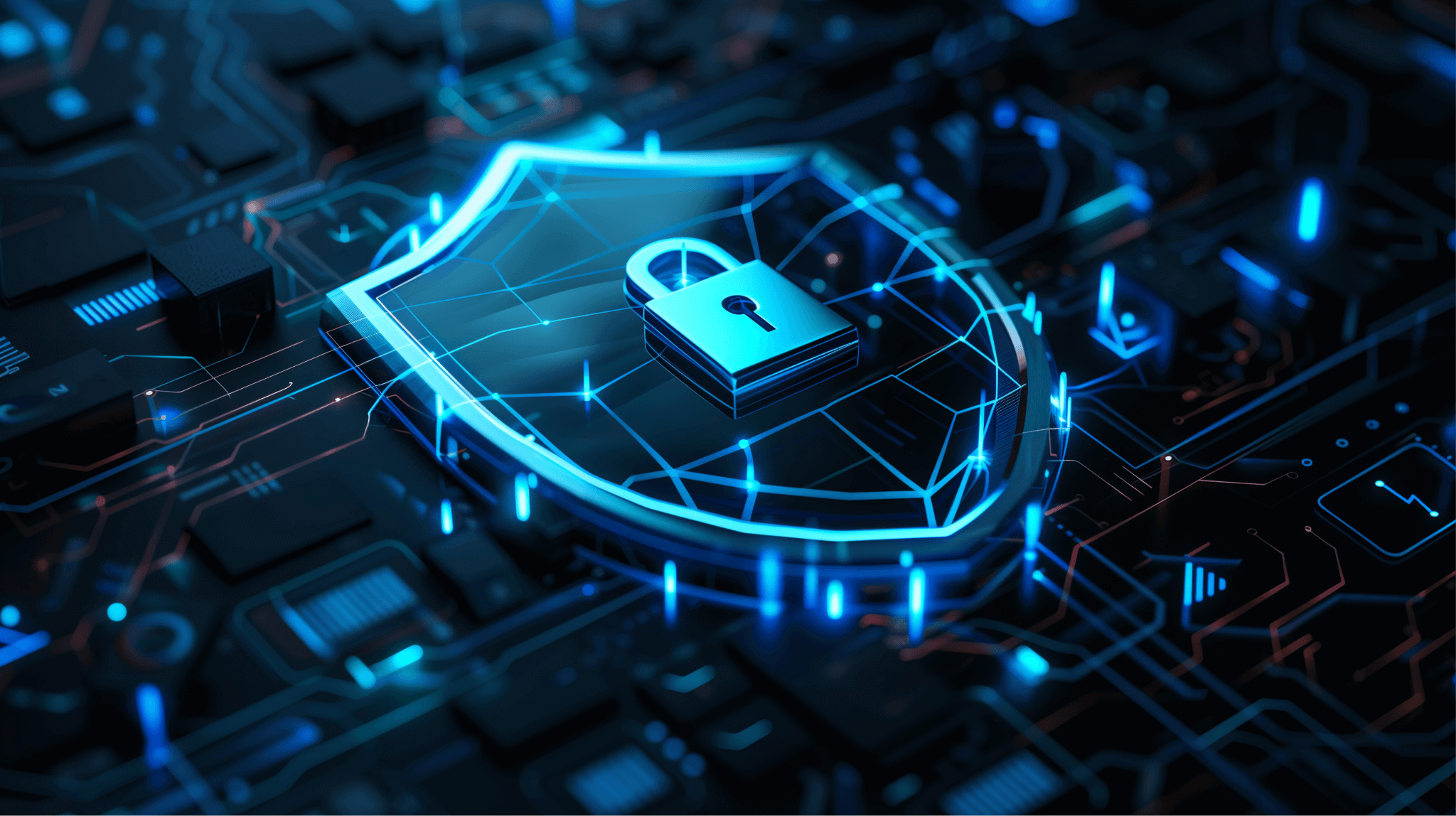
Let's get real: Malware, phishing attacks, data breaches—they're becoming more sophisticated by the day, leaving businesses scrambling to keep up.
But here's where things get interesting: generative AI in cyber security is completely changing the game in 2024. As cyber threats evolve at machine learning speed, organizations are turning to AI-powered solutions that can match—and exceed—this pace of evolution.
Imagine a security system that can simulate thousands of attack scenarios in seconds, automatically generate and deploy countermeasures, and continuously learn from every attempted breach. The impact is revolutionary. While traditional security tools rely on known threat signatures and manual responses, generative AI for cybersecurity is creating dynamic defense systems that adapt in real-time.
In this article, we'll dive into how generative AI in cybersecurity is transforming cybersecurity and why it's becoming the secret weapon for businesses serious about protecting their digital assets.
The Role of Generative AI in Cybersecurity
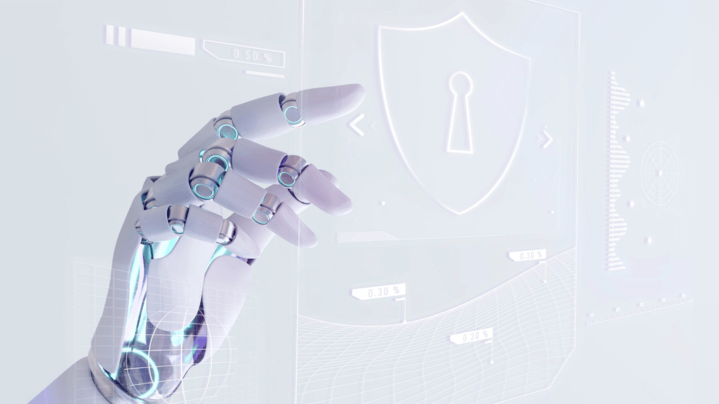
Unlike traditional security tools that operate on pre-defined rules and signatures, generative AI in cyber security brings something entirely new to the table: the ability to create, learn, and adapt to threats in real-time.
It does that by processing vast amounts of data from multiple sources to identify subtle patterns that human analysts might miss, powered by sophisticated deep learning algorithms.
From automatically generating and testing new security protocols to creating sophisticated threat detection models, generative AI in cybersecurity is handling tasks that would take human teams weeks to complete—in just minutes.
How Generative AI is Transforming Cybersecurity?
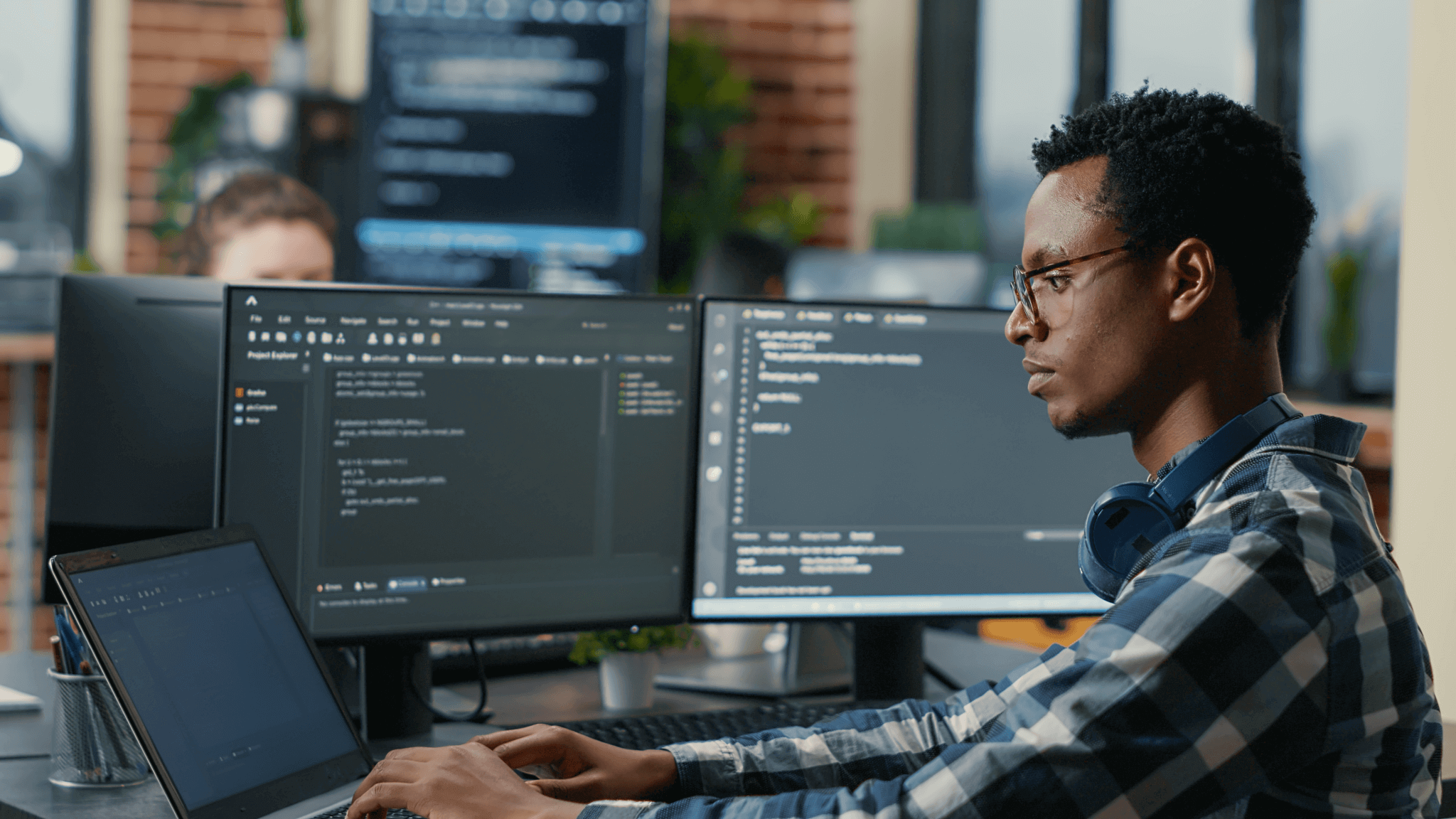
It's not just about speed, though; it's about the capabilities that weren't possible before. For instance, generative AI for cybersecurity can create synthetic datasets to train security systems, simulate complex attack scenarios, and even predict zero-day vulnerabilities before they're exploited.
This predictive capability is transforming how organizations approach their security posture. Rather than playing an endless game of catch-up, security teams can now implement preemptive measures through automated response systems and strengthen weak points before they're targeted.
The real magic happens when generative AI and cybersecurity combines with human expertise.
While AI handles the heavy lifting of data analysis and anomaly detection, human analysts can focus on strategic decision-making and complex problem-solving.
Benefits of Using Generative AI in Cybersecurity
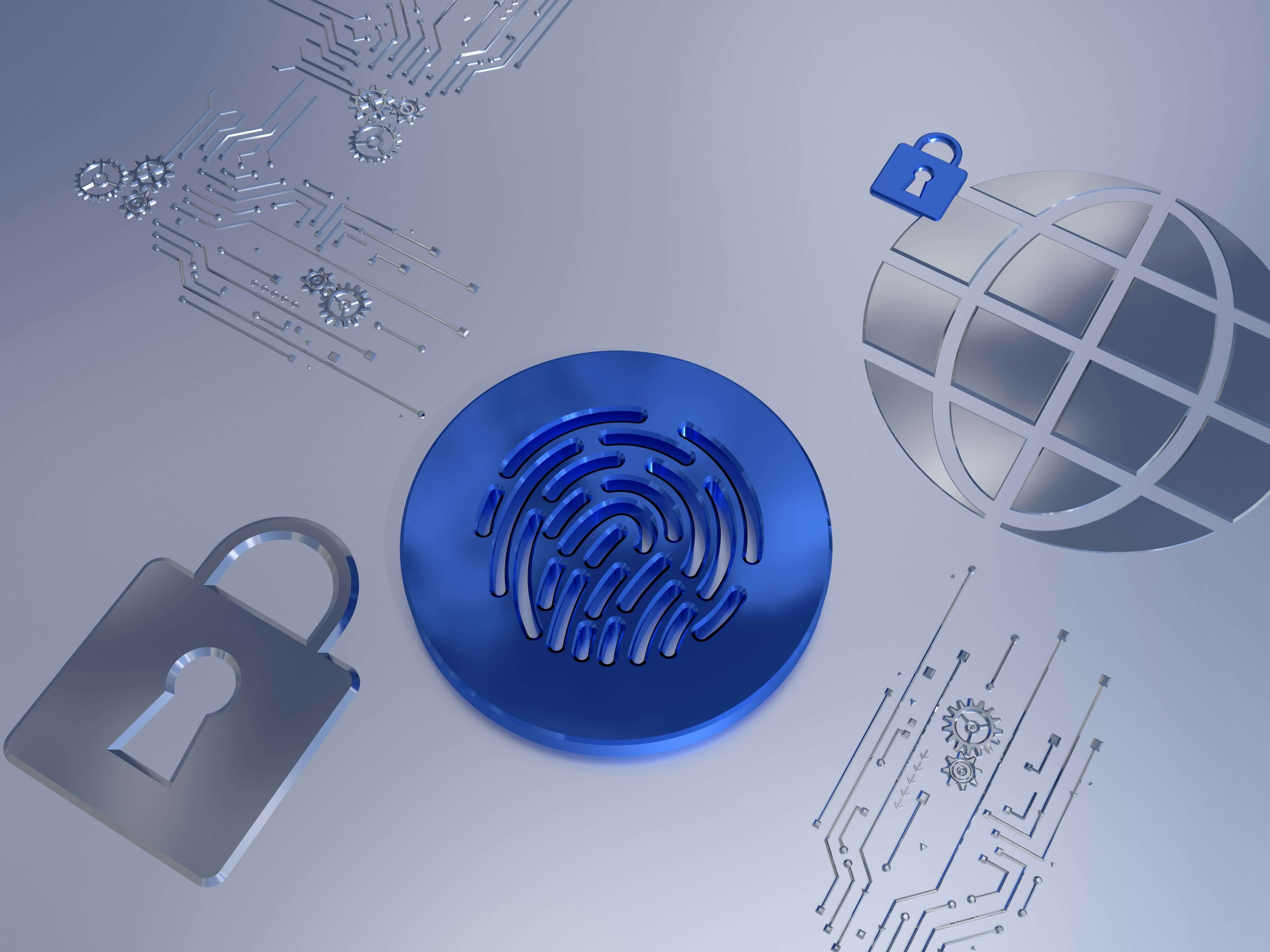
The integration of generative AI in cybersecurity infrastructure represents a paradigm shift in defensive capabilities.
Through the synergy of multiple AI technologies—including natural language processing (NLP), deep learning, and reinforcement learning—organizations can now implement proactive, adaptive, and autonomous security measures at scale.
The following benefits demonstrate how generative AI and cybersecurity is fundamentally transforming the landscape:
1. Predictive defense capabilities
Advanced neural networks and deep learning algorithms enable predictive modeling. By analyzing historical attack patterns, network behavior, and global threat intelligence feeds, generative AI constructs sophisticated prediction models that can forecast potential attack vectors weeks or months before they emerge.
- Leverages transformer architectures for threat indicator analysis
- Creates dynamic risk scoring models
- Analyzes dark web activities for emerging threats
- Predicts seasonal attack patterns
- Implements reinforcement learning for prediction refinement
2. Enhanced detection accuracy
Through multi-layered deep learning models and advanced malware identification systems, generative AI achieves unprecedented accuracy in threat detection. The system employs sophisticated feature extraction techniques and behavioral analysis to identify even the most subtle indicators of compromise, reducing false positives while maintaining high sensitivity to actual threats.
- Utilizes deep neural networks for encrypted traffic analysis
- Employs heuristic analysis for malware variants
- Uses graph neural networks for threat correlation
- Applies transfer learning across environments
3. Automated response systems
Automation frameworks supported by generative AI enable sophisticated incident response. The system employs decision trees and probabilistic models to determine optimal response strategies, while reinforcement learning ensures continuous improvement of response effectiveness.
- Deploys containerized security solutions
- Implements zero-trust segmentation
- Orchestrates responses across cloud environments
- Creates adaptive firewall rules
- Automates incident reporting
4. Resource optimization
Through sophisticated workload analysis and resource allocation algorithms, generative AI optimizes security operations across the entire infrastructure. Machine learning models predict resource requirements and automatically scale defensive capabilities, while intelligent task prioritization ensures critical security functions receive necessary resources.
- Implements predictive auto-scaling
- Uses optimization algorithms for resource allocation
- Employs ML-driven load balancing
- Optimizes CPU/GPU utilization
5. Continuous learning and improvement
Built on advanced neural architectures and self-supervised learning algorithms, the system continuously evolves its capabilities. Transfer learning enables rapid adaptation to new threats, while federated learning allows secure knowledge sharing across organizational boundaries without compromising sensitive data.
- Implements meta-learning for zero-day threats
- Uses reinforcement learning for policy optimization
- Employs active learning for training data
- Implements self-supervised learning
6. Comprehensive training and simulation
Generative AI creates realistic training scenarios and simulations that help security teams prepare for various cyber threats. These training environments adapt to emerging threats and provide hands-on experience in dealing with complex security challenges.
- Generates attack scenarios
- Creates threat simulation environments
- Produces synthetic training datasets
- Develops adaptive training programs
- Simulates breach scenarios
Challenges and Limitations of Generative AI in Cybersecurity
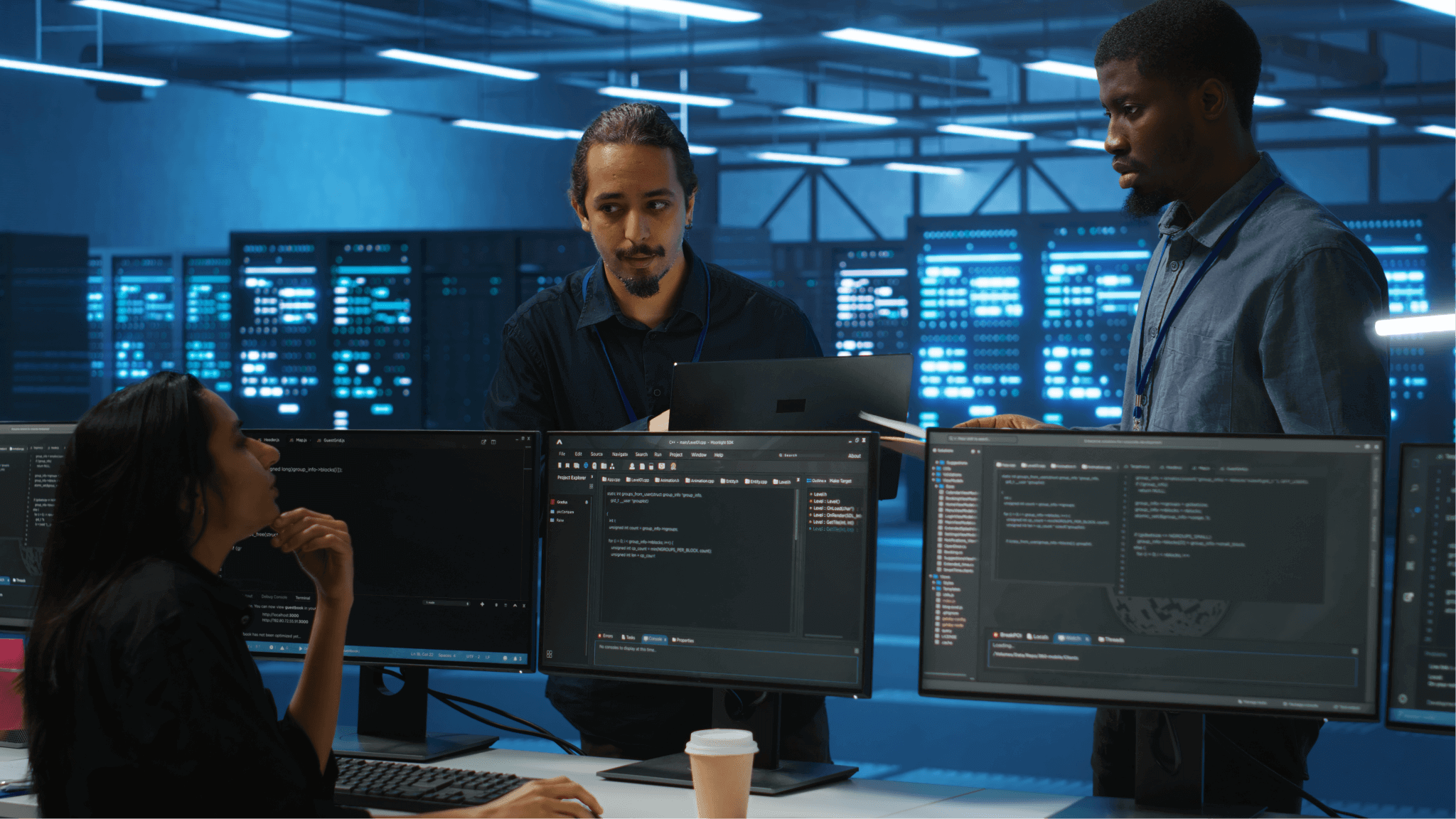
1. Quality control in AI training data
While generative AI in cybersecurity promises enhanced security, organizations must navigate important considerations around AI ethics and data privacy. Success requires balancing technological capabilities with regulatory compliance and ethical considerations.
- Training data contamination from poisoned sources
- Historical bias leading to missed emerging threat patterns
- Incomplete attack vector representation in datasets
- Validation challenges for AI-generated security scenarios
- Gaps in threat intelligence data coverage
2. Infrastructure and cost barriers
Beyond the initial investment, organizations must contend with the substantial ongoing costs and infrastructure demands of running sophisticated AI security systems. The resource requirements often exceed initial projections, creating unexpected budgetary pressures and operational challenges.
- Enterprise-grade GPU clusters for real-time threat analysis
- Massive storage requirements for security telemetry data
- High-bandwidth network infrastructure needs
- Continuous model training and optimization costs
- Specialized cooling and power infrastructure
3. Architectural complexity and integration
Implementing generative AI security solutions requires navigating a web of challenges, from integration with legacy systems to maintaining performance at scale. Organizations must balance the promise of AI capabilities with the practical limitations of their existing infrastructure.
- Legacy system compatibility issues
- Performance bottlenecks in real-time processing
- Complex microservice architecture requirements
- API integration and versioning challenges
4. Weaponization of AI
As organizations deploy AI for defense, adversaries are increasingly weaponizing the same technology for attacks. This creates an AI arms race where defensive systems must constantly evolve to counter AI-powered threats, leading to a complex and resource-intensive security landscape.
- AI-generated phishing content that bypasses filters
- Automated vulnerability discovery and exploitation
- Intelligent malware that evades detection
- Social engineering attacks using deepfake technology
- AI-powered password cracking and network penetration
5. Privacy and compliance
Organizations must balance the capabilities of AI security systems with data privacy requirements and evolving regulatory frameworks. This creates a complex compliance landscape where security objectives must be achieved within strict legal boundaries.
- GDPR compliance in AI data processing
- Cross-border data sovereignty issues
- Audit requirements for AI decision-making
- Regulatory reporting for AI security measures
6. Human-AI security integration
Successfully deploying AI security systems requires more than technical implementation—it demands a fundamental shift in how security teams operate. Organizations must develop new workflows that effectively combine human expertise with AI capabilities.
- Security team resistance to AI adoption
- Skill gaps in AI security operations
- Trust issues with AI-generated alerts
- Communication barriers between AI and human analysts
The Future of Generative AI in Security
While the challenges are significant—from data quality to infrastructure demands—the benefits of AI-powered security solutions are transformative. Organizations successfully implementing these systems gain unprecedented defensive capabilities that evolve alongside emerging threats.
At Liquid IT, we understand there's no one-size-fits-all approach to AI security implementation. We deliver customized generative AI and cybersecurity solutions that address your specific challenges.
Our team provides seamless integration with existing infrastructure, 24/7 monitoring, and comprehensive staff training, all while ensuring compliance with industry-specific frameworks through regular security audits and assessments.
Don't let the complexity of generative AI in cybersecurity hold you back from enhancing your security posture. Contact us today to learn how we can help your organization.